Artificial intelligence (AI) has increasingly been recognised and emphasised as an essential priority for individuals, organisations, and governments. More than ever before, academics, businesspeople, bureaucrats, and politicians alike have shown much interest in AI. In fact, many of these people believe that AI can play a critical role in improving performance, competitiveness, and economic wealth.
The tremendous attention paid to Al is reflected in the dramatic increase in books and research addressing AI’s importance to organizations. Despite the significant increase in the amount of literature on AI over the years, the literature in this field has yet been able to provide a more comprehensive perspective of AI. The review of previous literature on AI reveals that limited attempts have been made to emphasize and explain issues related to its nature, advantages, and limitations.
Artificial intelligence enables technical systems to conduct activities that require human intelligence, such as learning, problem-solving and decision-making. In computer science, AI develops machine learning (ML) models (Figure 1) that can learn to perform specific tasks. Over the years, as AI technology progressed to a more advanced level, it developed the improved foundation model (as illustrated in Figure 2), which replaced the task-specific models. The foundation model can perform a range of general-purpose tasks.
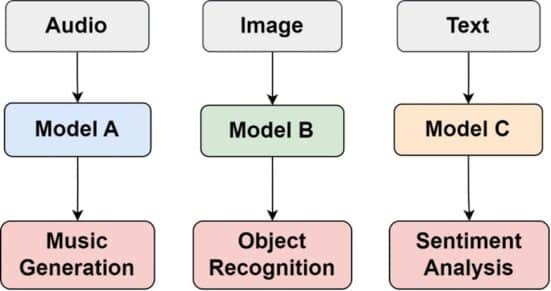
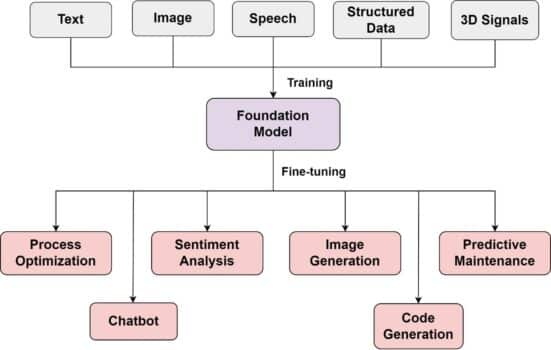
Recently introduced advanced generative artificial intelligence models included the ChatGPT-4, Llama 3, DALL.E 3, and Gemini 2.0. These models have changed the way technical systems produce user content. In addition, they have changed how we work, shop, communicate and revolutionized industries such as software, creative, travel and supply chain. For example, Google Gemini can assist in improving the productivity of the software development industry by generating, debugging, and explaining the program code in different programming languages. In the creative sector, the Adobe Firefly AI tool can also boost the creative process of graphic designers in producing custom artwork by generating high and realistic images by prompting text descriptions.
Generative AI tools can enhance the customer experience for online travel agencies by generating personalized recommendations based on individual preferences. For instance, based on the customer’s historical travel data, Booking.com can recommend real-time travel updates for destinations, activities, and accommodations [3]. Aviation businesses can also integrate chatbots into their websites to handle flight bookings and passengers’ inquiries, provide airline schedules, and issue alerts for schedule disruptions.
Generative AI can also help to optimize supply chain businesses [4]. For example, fuel consumption costs can be reduced by designing efficient picking and delivery routes for drivers based on traffic conditions and the priority of deliveries. Predictive maintenance can determine which machines or instruments are most likely to fail. This allows manufacturers to schedule maintenance efficiently and improves overall equipment effectiveness (OEE).
Although GAI can provide various advantages as a technology, it has several limitations related to the accuracy of its generated content. For instance, the generated content can be false or misleading but presented as facts to the users. These misleading contents are known as hallucinations [5]. For example, ChatGPT can generate fictional citations for an article that does not correspond to authentic sources. This hallucination occurred due to insufficient training data and biases in data used to train the generative AI model. As such, it is crucial not to rely solely on the generated results before reliable sources verify them to ensure factual accuracy.
While generative AI can provide solutions and complete tasks quickly, it cannot help develop critical thinking skills. Overreliance on generative AI tools can also cause cognitive atrophy, which can be detrimental to individuals’ effective learning process in the long term. For example, students are often given sample articles to read and learn to develop classroom writing skills. However, if they rely on the ChatGPT to complete their writing assignments [6], they cannot develop their writing skills.
Equally important, generative AI raises significant ethical concerns regarding the potential misuse or manipulation of the generated content that may unintentionally or intentionally defame individuals or organizations. For example, realistic videos (deepfakes) can be generated to make it appear that a specific individual said or did something they never did [7]. In addition, malicious content such as fake news or social media posts can also be generated to spread misinformation or propaganda that can influence public opinion.
The training and utilization of the generative AI model involve the use of robust hardware and significant electrical energy. Recently, it has been found that training and utilizing generative AI models can have negative environmental impacts on the planet regarding their carbon dioxide emissions. According to the study by [8], generating 1,000 images with Stable Diffusion XL can contribute to carbon dioxide emissions roughly equal to travelling 4.1 miles in a typical gasoline-powered car.
In summary, although generative AI has the potential to increase productivity, generate income, and bring positive changes to the way we work, shop, and communicate, it is also known that this technology can negatively influence our learning and working environment. While generative AI and its tools can enhance certain aspects of our work, they still lack the general intelligence, creativity, and social awareness that humans possess. To maximize the benefits and mitigate the risks of adopting the GAI, its adoption must be accompanied by proper skill development strategies, ethical governance, and quality assurance mechanisms, and it must be embedded with values such as fairness and accountability.
References
- NVIDIA, “Generative AI – What is it and How Does it Work?,” NVIDIA. https://www.nvidia.com/en-us/glossary/generative-ai/
- Merritt, “What Are Foundation Models?,” NVIDIA, Jan. 24, 2024. https://blogs.nvidia.com/blog/what-are-foundation-models/
- L. Jockims, “ChatGPT and generative A.I. are already changing the way we book trips and travel,” CNBC, Apr. 22, 2023. https://www.cnbc.com/2023/04/22/how-chatgpt-generative-ai-are-changing-how-we-book-trips-and-travel.html
- Steinberg, “How supply chains benefit from using generative AI,” Ernst & Young, Jan. 9, 2024. https://www.ey.com/en_gl/insights/supply-chain/how-generative-ai-in-supply-chain-can-drive-value
- Cornell University, “Generative Artificial Intelligence,” Cornell University Centre for Teaching Innovation, 2024. https://teaching.cornell.edu/generative-artificial-intelligence
- Feijo and K. Ouellette, “What will the future of education look like in a world with generative AI,” MIT Open Learning, Dec. 18, 2023. https://openlearning.mit.edu/news/what-will-future-education-look-world-generative-ai
- Chan and A. Swenson, “Opinion: How to spot AI-generated deepfake images,” The Star, Mar. 21, 2024. https://www.thestar.com.my/tech/tech-news/2024/03/21/opinion-how-to-spot-ai-generated-deepfake-images
- S. Luccioni, Y. Jernite, and E. Strubell, “Power Hungry Processing: Watts Driving the Cost of AI Deployment?,” arXiv.org, Nov. 28, 2023. https://arxiv.org/abs/2311.16863