Artificial intelligence (AI) has become a transformative technology in our daily lives. Since its introduction, AI has provided innovative solutions to various problems facing modern society. The technology has been rapidly adopted in many economic sectors, including healthcare, education, finance, banking, and law enforcement.
However, a recent survey conducted by the University of Queensland and Klynveld Peat Marwick Goerdeler (KPMG) Australia revealed that three out of five people (61%) across 17 countries are unwilling to trust AI systems. Additionally, three out of four respondents (73%) expressed concerns about potential risks associated with AI, such as loss of privacy, deterioration of human rights, and inaccurate outcomes. These findings highlight not only the apprehensions surrounding AI adoption but also indicate a significant need to strengthen public trust in the technology through the adoption of responsible AI practices.
As AI continues to evolve, it presents a dual challenge: it is both a valuable tool and a source of concern. While organizations employ AI to address complex problems, they also face the challenge of harnessing its transformative power responsibly. As AI systems become increasingly complex and capable, it is crucial to ensure they are operated ethically and safely alongside human users. This calls for the development of more responsible AI systems.
Responsible AI seeks to integrate ethical and safety principles into these systems, thereby mitigating risks and adverse outcomes while maximising their potential benefits. Moreover, responsible AI focuses on accountability and compliance, which can positively impact the use of AI-powered systems.
Over the years, numerous efforts have been made to establish key principles, frameworks, and standards for responsible AI practice. Generally, the focus of responsible AI has been confined to four important areas: explainability, fairness, robustness, and privacy.
Explainability refers to the capability of AI systems to provide explanations for their decisions. For instance, the one-class fully convolutional data description (FCDD) anomaly detection network has been utilized to generate visual explanations, helping human observers understand why the model classifies pill images as normal or defective. Figure 1 illustrates the anomaly heat maps on pill images.
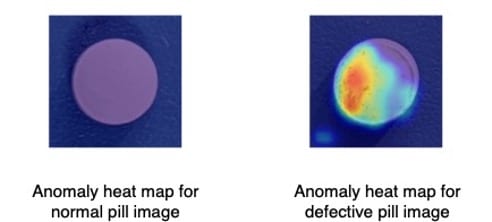
Fairness involves ensuring that AI systems deliver just and equitable outcomes for individuals or groups, regardless of factors such as gender, age, or race. For instance, when AI systems provide recommendations on topics like medical treatment, loan applications, or employment, they should avoid bias and make consistent suggestions for individuals or groups with identical symptoms, financial situations, or qualifications.
Robustness refers to the ability of AI systems to maintain their performance in challenging conditions. For example, a self-driving vehicle that lacks robustness may be misled into thinking a stop sign is absent if a malicious party deliberately obscures part of it using adversarial attacks. Figure 2 illustrates an adversarial attack on a stop sign.

Privacy entails safeguarding the personal information within AI systems from unauthorized access or misuse that could harm individuals or organizations. A notable example is the British consulting firm Cambridge Analytica, which collected personal data from millions of Facebook users without their consent for the purpose of developing political advertising.
Evidence suggests that integrating responsible AI approaches has a significant impact on fields such as law enforcement, healthcare, and finance. For instance, the Axon company advocates that police officers should be trained to recognize that AI cannot fully replace human decision-making. While AI systems can analyze body-worn camera (BWC) recordings to identify instances of police misconduct, supervisors should not rely solely on these outputs. They must personally review flagged recordings before making any final recommendations.
The adoption of responsible AI practices is equally crucial in the healthcare sector. The pharmaceutical company Pfizer emphasizes that stakeholders, including data scientists and engineers, must ensure the use of unbiased data and algorithms when developing AI systems in this sector. If algorithms are trained on limited datasets, the resulting AI outputs may not accurately represent the broader population, potentially leading to an unequal distribution of healthcare services.
Responsible AI practices must also be implemented in the finance sector. According to the Focus People company, the decisions and outcomes generated by AI models in financial organizations must be transparent and explainable. The AI decision-making processes should be documented, regularly audited, and reviewed. Such measures will not only build customer trust but also ensure compliance with regulatory requirements.
In conclusion, the widespread adoption of AI models creates a pressing need for the industry to take greater responsibility for the negative impacts of this technology. The benefits that AI can bring to society can only outweigh its adverse effects if the AI industry collectively embraces responsible practices that prioritize explainability, fairness, robustness, and privacy. Responsible AI practices can enhance the reliability and validity of AI systems while also fostering trust and accountability among human users.